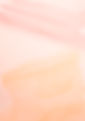
Youtube Bias Tracker
User-Centered Research and Evaluation
Role
Project Type
Timeline
Target Audience
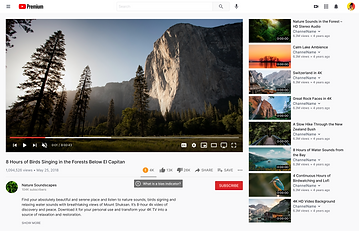
Project Manager (Main)
UX Researcher/Designer
October 5th, 2021 - December 8th, 2021
Product concept development based on user research
Everyday users of technology, who actively use and express their opinions on Youtube
Project Details
Tools
Group project: 5 members
Miro (brainstorming and diagramming)
Figma (product development)
Google Docs (documentation)


What is this project?
Opportunity
Our project and research were based on the goal of mitigating and eventually auditing algorithmic bias in technology. We researched how we can utilize the power of users and their everyday habits, patterns, and pain points with technology to create a tool that would encourage users to promote unbiased content and/or warn other users and the platform they are using about any problematic content being suggested to them. This would allow users to be in control of what they see on social media, online streaming platforms, etc. and consequently reduce the occurrence of bias. We chose to focus on Youtube as our target platform, and talked to users to find ways to stimulate healthy online engagement and feedback.
Solution
With insights from our research, we came up with a new YouTube metric – a Bias Indicator. People can click this button to easily provide their opinion about the biases that appeared in the video. If enough users indicate that the video is biased, the video will be taken down. This button not only helps the platform track problematic videos to update Youtube’s recommendation algorithm, but also provides users transparency about other users’ opinions by color-coded indicators (red, orange, green) and the number of reports. The bias indicator also comes with clear and concise documentation that informs users about the appropriate context to use the indicator while reassuring them about the efficacy and impact of their actions.

Problem Space
Project context, research focus, and target audiences
The problem space consisted of the effects of algorithmic bias on online and real-life communities, and how this bias can translate into harmful consequences of technology. Our research and study was an important start to create awareness of algorithmic bias, and gain insights into how everyday technology users are affected by this bias and if/how they respond to it. We define bias as prejudice in favor of or against one thing, person, or group compared with another. This algorithmic bias can be implicit but harmful to many populations through racial discrimination, inequality, targeting mental health, invasion of privacy, etc.
We aimed to create a tool that would target algorithmic bias through users and help mitigate and eventually audit algorithmic bias through the general use of social platforms. The purpose of the study was to understand how to motivate users to express their views in an actionable, trackable way that would affect algorithmic bias.
Our goal was to make the process of online engagement and interaction feel safer, more encouraging, and accommodating for everyday users of technology. Our main goals included:
1
2
3
Encourage users to promote unbiased content and/or warn other users and the platform of problematic content
Provide accessible bias-auditing actions and transparency about the impact of user actions
Stimulate healthy online engagement and feedback to mitigate and eventually audit algorithmic bias
Based on our goals, we developed a few different “How might we” statements, which we then narrowed down to:
How might we utilize user engagement and expression to mitigate
algorithmic bias in technology?
We also further defined our problem space and possible solutions that could be crafted with research methods:
Area of Focus
Informing our users of the impact of bias in their reporting/audit algorithms and reassuring them that their identification of bias will have some tangible impact
Context
Digital spaces like search engines (e.g. Google Search), social media platforms (e.g. Youtube, Twitter, Instagram)
Possible Tools/Products
Plug-ins, Indicators, APIs, Visualizations, Online surveys

User Research
Background, generative, and evaluative research methods
To understand how to audit algorithmic bias on Youtube and social media, we conducted a variety of generative and evaluative research methods such as think-aloud study, contextual inquiry, directed storytelling, affinity mapping, and speed dating. Through these research methods, we were able to create a solution to fit our users’ needs.
Preliminary
Background Research
Data Analysis
Semi-structured interview
Define
Synthesize, Ideate & Define
Worst Idea Possible
Defining Research Goals
Ideation
Think-Aloud Protocol
Contextual Inquiry
Affinity Diagramming
Empathy Mapping
Evaluation
Interpretation
Storyboarding
Scenarios
Speed Dating

The insights and evidence we gained through these methods helped us develop ideas for our final design and also critique them with an audience that would actually use these ideas if made public. This process made our final product much more human-centered and catered towards use cases rather than focusing on aesthetics.

Research Evidence
User study methods and results
Through our research methods, we were able to get direct input like quotes from users within our problem space about encountering problematic content online, interacting with that content beyond just viewing, and how they deal with disliking, reporting, and/or creating awareness about biased content on content sharing platforms.
The following is evidence collected through interviews, think-aloud protocols, and speed dating sessions:
1
Our user interviews showed that users have different reasons for disliking videos.
I would only dislike a video if a friend sent it to me and it was stupid and a waste of time. I would dislike it out of spite
"
"
I only dislike video that is spreading misinformation or low-quality
"
"
I only uses the dislike button if I’m not interested or think it's harmful
"
"
2
Because of the nature of social media, users often just scroll and not take actions.
I ignore a lot of content since a lot of posts on social media is rough for my mental health
"
"
If I see a problematic video from channels on my recommended videos, I just think the channel is stupid. I won’t do anything about it
"
"
If I encounter a problematic video, I would only report it if it’s fundamentally wrong
"
"
3
People expect reporting to have an effect
I just hope that enough people report it to get it taken down
"
"
I see reporting directly to the platform as a way to inform the algorithm
"
"

Research Insights
Research evidence-based insights, relevant pain points, etc.
We organized the evidence we collected into groups of possible insights that could be drawn from users. These insights boiled down into the following relevant pain points. We targeted these pain points when we ideated and tested our design solution.
There is no consistent use and purpose behind disliking, reporting, and marking as “not interested”
Disliking, reporting, and/or flagging actions fail to serve as a consistent and reliable metric for gauging harmful content on Youtube. From our speed dating sessions, users understood the significance and preferred guidelines distinguishing the uses of each of these actions and documentation informing them about the outcomes of these actions (whether that be informing the algorithm, potentially removing the video from YouTube, etc.).
Users want speed and convenience when reporting bias in a video.
Users are primed on social media to scroll past instead of engaging with problematic biased content. As such, from our speed dating sessions, we found that users had a high affinity for quick, easily accessible actions that allowed them to flag or report harmful/ostracizing videos. For example, using the like/dislike button on Youtube was more preferred because it was quick and easy for users to provide feedback, rather than going through a questionnaire to report a video.
Users want an assurance of efficacy and impact following their actions.
Users want to know that their actions are having an effect. The effect of their actions, however, are often unclear to them due to a lack of documentation and feedback. Having transparency of the effect of their actions provides a sense of mental satisfaction that their complaints/opinions are being attended to and possibly solved.

Final Solution
Conceptual solution and visual mockups
Our solution, a new Youtube metric button ‘Bias Indicator’ is accessible similar to like/dislike, comment, etc. buttons, but will measure biased content in a video. Users can click on the button to provide their feedback, which will then be analyzed by Youtube to identify and filter bias videos. This indicator is useful not only for users to express their opinions but also for Youtube’s algorithm to understand when a video is biased, and when it hits a certain threshold of yellow/red indicators, the video would be hidden or taken down for harmful content.

Bias Indicator button, located to the left of the like/dislike buttons: keeping the button accessible to all users viewing videos
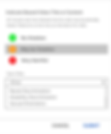
Using the bias indicator button:
The button intends to collect data from users in the form of three color-coded metrics: green for no bias, yellow for possibly hurtful bias, and red for extremely biased or harmful content

Documentation:
Provides instructions about what is bias, when to use, and how to use the bias indicator
Our solution allows users to safely engage with online content while creating awareness of algorithmic bias through people’s everyday online expression and usage. We aimed to provide accessible options to audit videos, provide transparency of actions, and harness users’ ability to interact with content as a tool to empower them to mitigate algorithmic bias.